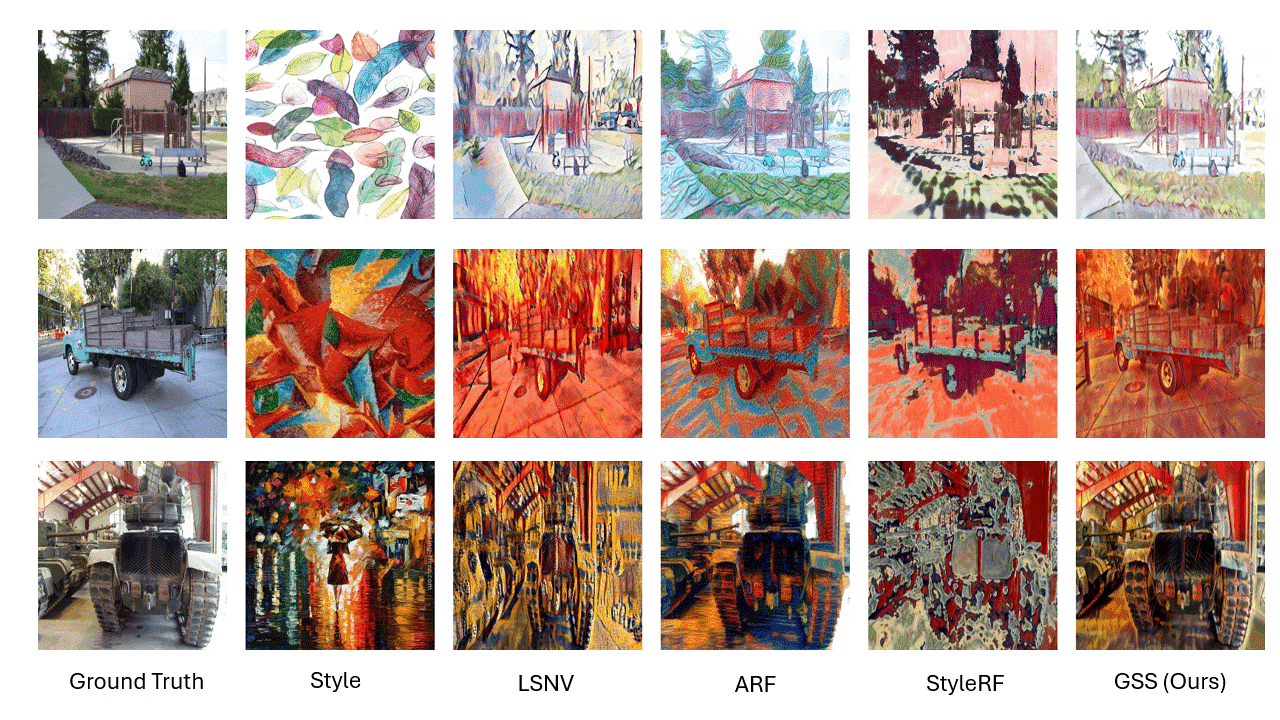
Gaussian Splatting in Style
We are the first to employ Gaussian Splatting to solve the task of scene stylization, extending the work of neural style transfer to three spatial dimensions.
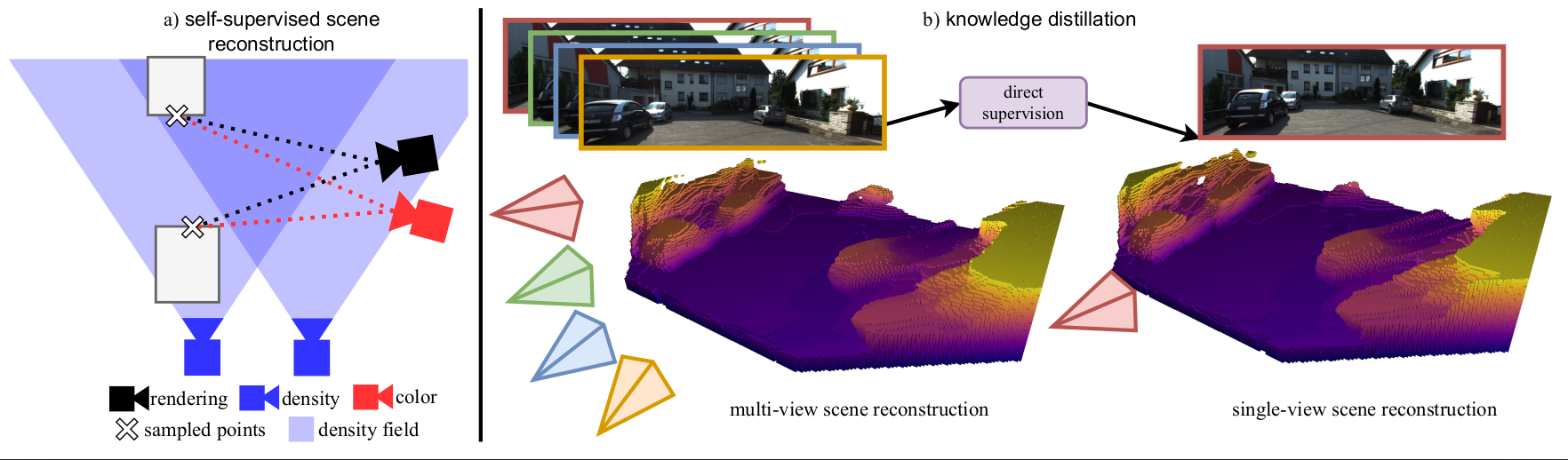
Boosting Self-Supervision for Single View Scene Completion via Knowledge Distillation
We use multi-view scene completion to supervise single-view scene completion and boost its performance. We propose both a novel multi-view scene completion network and a corresponding knowledge distillation scheme.
Project Page | Paper | Code | Citation
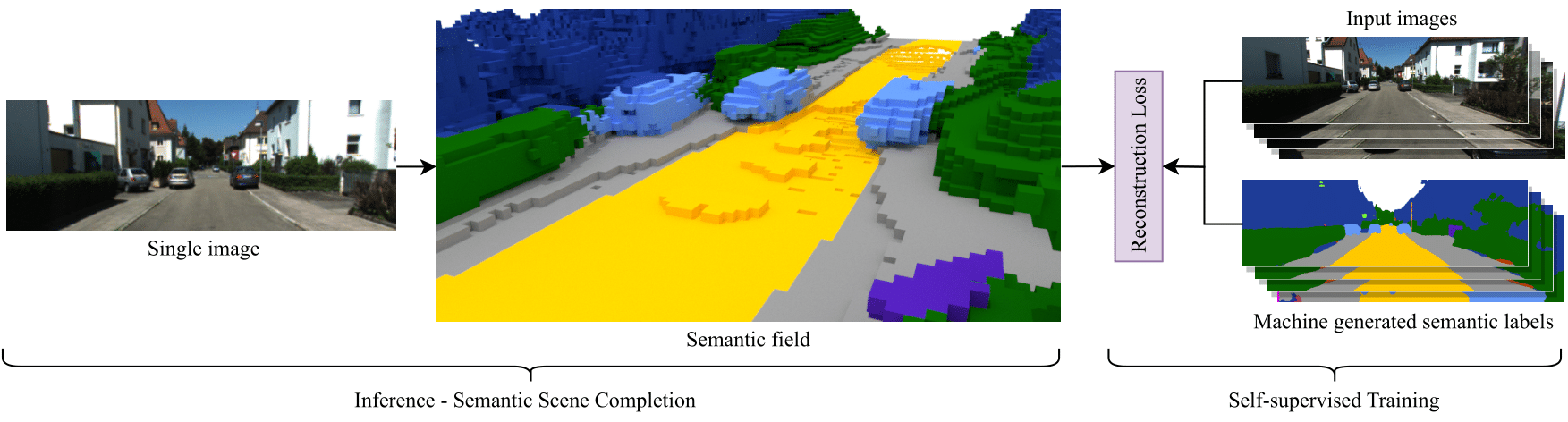
S4C: Self-Supervised Semantic Scene Completion with Neural Fields
S4C is the first self-supervised approach to the Sematic Scence Completion task. It achives close to state-of-the-art performance on the KITTI-360 SSCBench dataset.
Project Page | Paper | Code | Citation
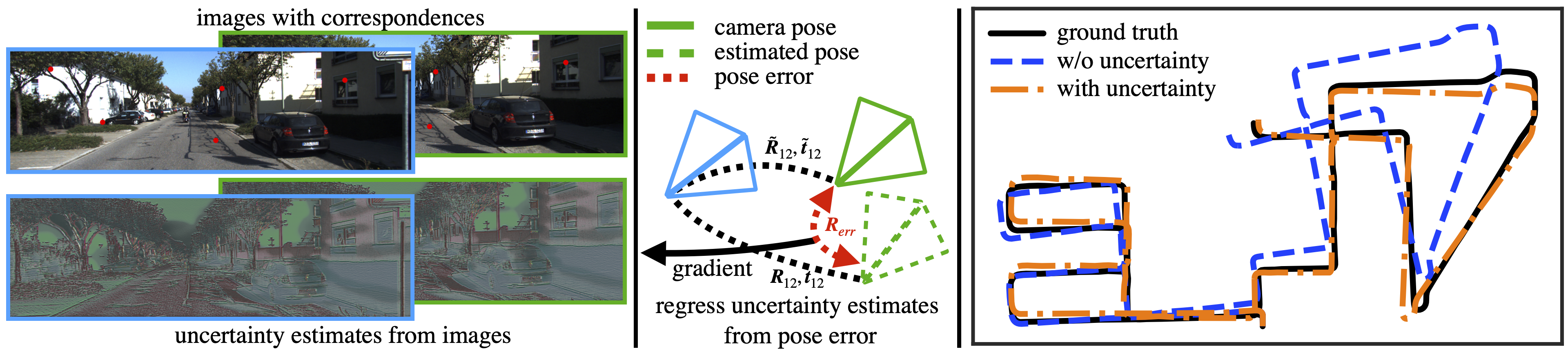
Learning Correspondence Uncertainty via Differentiable Nonlinear Least Squares
A differentiable nonlinear least squares framework to account for uncertainty in relative pose estimation from feature correspondences regardless of the feature extraction algorithm of choice.
Project Page | Paper | Code | Video | Citation
Probabilistic Normal Epipolar Constraint for Frame-To-Frame Rotation Optimization under Uncertain Feature Positions
We propose a probabilistic extension to the normal epipolar constraint (NEC) which we call the PNEC. It allows to account for keypoint position uncertainty in images to produce more accurate frame to frame pose estimates.
Project Page | Paper | Code | Video | Citation